One of the most attractive parts of digital marketing is the built-in focus on data.
If a tactic tends to have positive data around it, it’s easier to adopt. Likewise, if a tactic hasn’t been proven, it can be tough to gain buy-in to test.
The main way digital marketers build that data confidence is through studies. These studies typically fall into one of two categories:
- Anecdotal: A limited number of data points, however, there’s typically far more detail on the individual mechanics.
- Statistically significant: A large number of data points (typically 100+) that might be forced into more simple analysis due to the sheer volume of entities being analyzed.
Both data sets have their place in building out digital marketing strategies. This is why it’s dangerous to lean too heavily into one or the other.
As someone who has worked within organizations capable of putting out both types of data sets – and an avid consumer of both – I thought it would be useful to dig into:
- Minimum criteria for each type of study.
- What value brands can get out of both types of studies.
- How to set up your own studies.
This post will look at a few different studies ranging in digital marketing disciplines.
This is because the core principles that govern anecdotal (smaller data) and statistically significant (big data) are fairly similar across marketing disciplines.
Minimum Criteria For Each Type Of Study
A common mistake folks make when setting up studies is thinking the volume of data is the only criterion to make their studies valuable.
Yes, it is lovely when there’s a lot of data, but there are other critical factors:
- How many variables are being considered?
- What, if any, mitigation is there for outliers/excess variables?
- Can the study respond to critics with data vs. emotion?
These three will be minimum requirements regardless of whether you focus on an anecdotal study or a statistically significant one. However, there are some study-specific criteria as well.
Anecdotal Studies
When looking at a smaller data set (i.e., fewer than 10 accounts, less than a year of data, etc.), there’s much more pressure to dig into the before and after impacts of whatever thing you want to test.
People will want as much detail as possible because the study usually shows the results of specific actions taken in one account/for one brand.
This means screenshots will be critical. If you can’t show exactly what happened, it won’t be taken seriously.
However, screenshots do not require you to reveal the client you’re working for. Filtering out brand names is absolutely reasonable.
Leaving out benchmarks, important metrics, and whether an initiative had “unfair advantages” (big budget, branded campaigns, etc.) is not.
A good example of an anecdotal study is looking at the impact of a change over a few months. This graph from Will O’Harra shows the shift in site traffic for “fan” sites vs. big names.
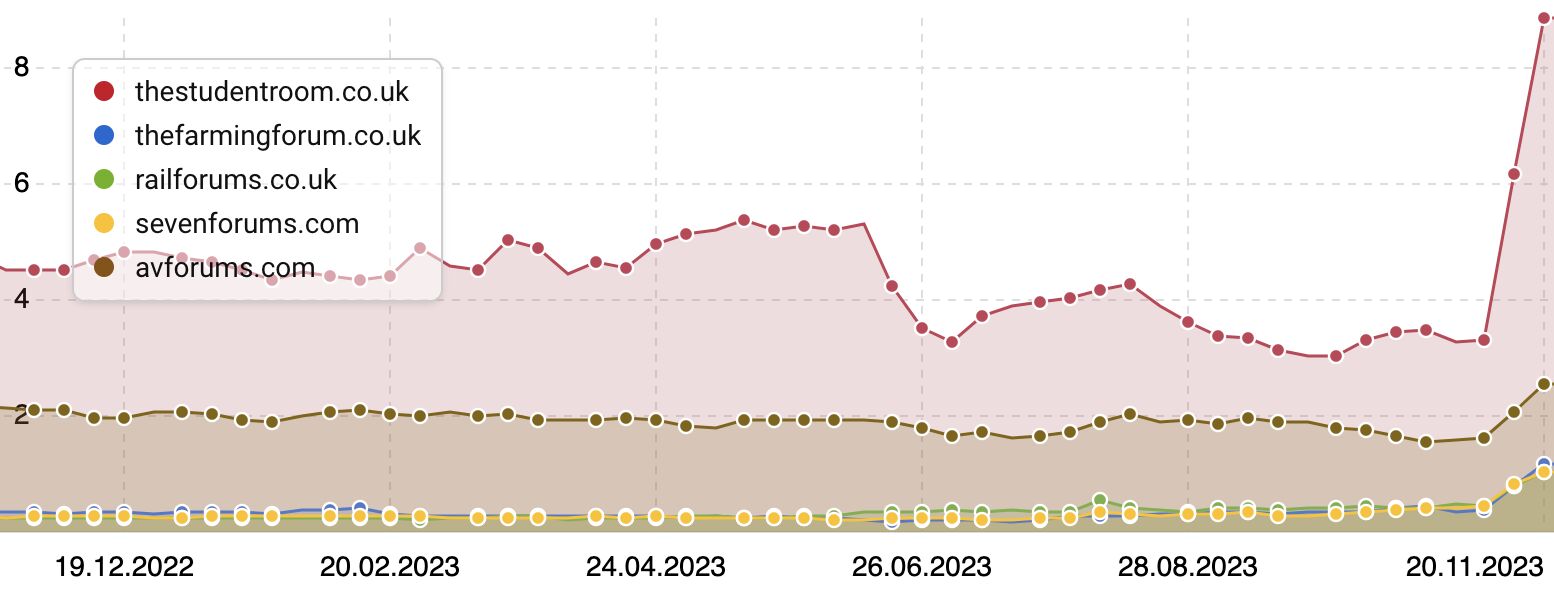
In this study, we can see sites that would otherwise have lower traffic getting a big spike due to the change in quality content criteria. This is an anecdotal study in that it only looks at five sites.
Big Data Studies
Where folks will be fairly unforgiving of the lack of detail in anecdotal studies, big data studies get a little more leniency.
This is because their main measure is the volume of accounts that speak to a specific trend. However, this doesn’t mean big data studies are free from scrutiny – just that the focus is on different things.
Big data needs to be very stringent in inclusion criteria. Entities included need to be as close to each other as possible.
Additionally, big data studies typically need a lot of entities. If you’re going to make a comment about a particular trend, there needs to be enough volume to back up the claim.
For example, in my Optmyzr study looking at Google match types and bidding strategies, we included roughly 2,600 accounts across multiple countries. (Disclaimer: I work for Optmyzr.) We could have included more accounts if we were more lenient on the criteria.
What Value Brands Can Get Out of Both Types Of Studies
It can be tempting to only focus on one type of study. However, both have their place and can inform meaningful account strategy.
Big data is helpful to understand overarching concepts and trends that can impact your account. These will be the guiding principles, like:
- Which structure choices have a higher chance of success?
- Where to focus content generation efforts.
- How are people spending their money?
- When to use which type of messaging in the buyer funnel?
What’s useful about these sorts of learnings is that they give you a good starting place for forming your strategy. They also can be useful to sanity check yourself.
For example, the brilliant Mike Ryan (SMEC) conducted a study on how many conversions are needed for successful PMax campaigns. While this data is useful in every context, knowing it’s based on 14,000 campaigns is helpful.
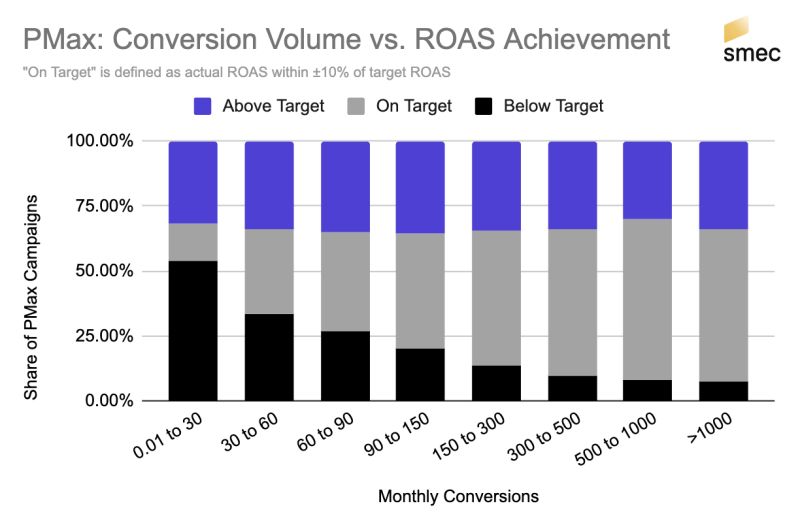
From this data, we can see that in order to achieve decent results, our PMax campaigns should be getting at least 60 conversions in a 30-day period.
If they can’t, it might be worth evaluating other campaign types. It’s very possible an account can succeed outside the results of this study, but they would be outliers to the general rule.
Similarly, the equal parts clever and entertaining Greg Gifford (Search Labs) did a study on Google Business Profile listings to evaluate if “best practices” actually hold up to analysis.
He and his team looked at 1,000 dealerships and found some best practices held true, while others were correlation instead of causation.
Anecdotal studies will be better at giving you “wild and crazy ideas” to test. They’re also very good for risk-tolerant folks to explore emerging trends.
How To Set Up Your Own Studies
Setting up studies comes down to understanding what the scope of the study will look like and how repeatable it is. If you only do a study once, it’s not as useful because trends are always shifting.
Additionally, if your scope is too narrow or wide, you might muddy the data or not fully address the important question.
Ensure that your hypothesis leaves room for you to be proven wrong.
If you don’t take precautions, data can be made to say anything. It’s critical to maintain strict guidelines of what is included and why.
More resources:
Featured Image: Sergey Nivens/Shutterstock